2020/10/16(Fri) 14:20 - 黃文良 博士 Dr. Wen-Liang Hwang (中央研究院資訊科學研究所 Institute of Information Science, Academia Sinica) - Un-rectifying Non-linear networks and optimizations
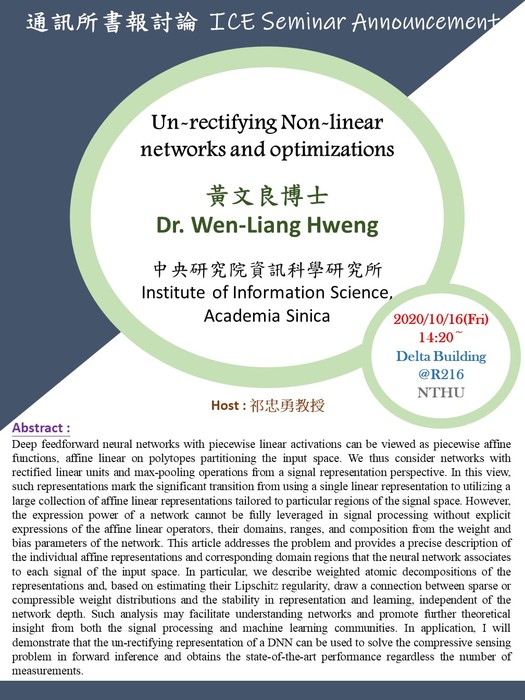
Date&Time :
2020 / 10 / 16 (Fri) 14:20 ~
Location :
Delta Building R216
Speaker :
黃文良 博士 Dr. Wen-Liang Hwang (中央研究院資訊科學研究所 Institute of Information Science, Academia Sinica)
Topic :
Un-rectifying Non-linear networks and optimizations
Abstract :
Deep feedforward neural networks with piecewise linear activations can be viewed as piecewise affine functions, affine linear on polytopes partitioning the input space. We thus consider networks with rectified linear units and max-pooling operations from a signal representation perspective. In this view, such representations mark the significant transition from using a single linear representation to utilizing a large collection of affine linear representations tailored to particular regions of the signal space. However, the expression power of a network cannot be fully leveraged in signal processing without explicit expressions of the affine linear operators, their domains, ranges, and composition from the weight and bias parameters of the network. This article addresses the problem and provides a precise description of the individual affine representations and corresponding domain regions that the neural network associates to each signal of the input space. In particular, we describe weighted atomic decompositions of the representations and, based on estimating their Lipschitz regularity, draw a connection between sparse or compressible weight distributions and the stability in representation and learning, independent of the network depth. Such analysis may facilitate understanding networks and promote further theoretical insight from both the signal processing and machine learning communities. In application, I will demonstrate that the un-rectifying representation of a DNN can be used to solve the compressive sensing problem in forward inference and obtains the state-of-the-art performance regardless the number of measurements.
Short Bio :
Wen-Liang Hwang received the B.S. degree in nuclear engineering from the National Tsing Hua University, Hsinchu, Taiwan, the M.S. degree in electrical engineering from the Polytechnic Institute of New York, New York, NY, USA, and the Ph.D. degree in computer science from New York University, New York, NY, USA, in 1993. He worked as a post doc with Professor R. Carmona. He is currently a Research Fellow in the Institute of Information Science, Academia Sinica, Taiwan. He is a co-author of the book Practical Time-Frequency Analysis, (Academic press, 1998). His research interests include deep neural network analysis and algorithm, computational mathematics, and large scale optimization.
In 2001, he received the National Award for Distinguished Junior Researchers in Taiwan. He is in the list of the ISI Highly-cited scholar in 2008 due to the work with his advisor S. Mallat on singularity detection and characterization with wavelets, published in 1992.