2024/4/12 (Fri.) 14:20- 謝秉鈞 教授 國立陽明交通大學 資工系 - Rethinking Non-Convex Optimization Through Reinforcement Learning
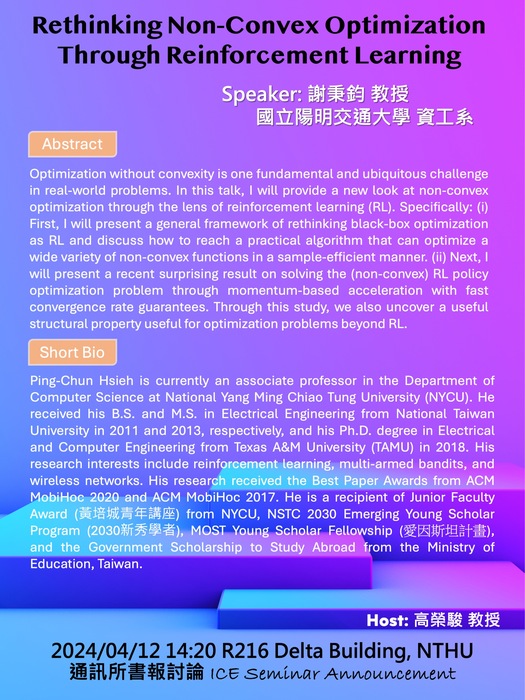
Date & Time:
2024 / 04 / 12 (Fri) 14:20 - 16:20
Location:
Delta Building R216, NTHU
Speaker:
謝秉鈞 教授
國立陽明交通大學 資工系
Topic:
Rethinking Non-Convex Optimization Through Reinforcement Learning
Abstract:
Optimization without convexity is one fundamental and ubiquitous challenge in real-world problems. In this talk, I will provide a new look at non-convex optimization through the lens of reinforcement learning (RL). Specifically: (i) First, I will present a general framework of rethinking black-box optimization as RL and discuss how to reach a practical algorithm that can optimize a wide variety of non-convex functions in a sample-efficient manner. (ii) Next, I will present a recent surprising result on solving the (non-convex) RL policy optimization problem through momentum-based acceleration with fast convergence rate guarantees. Through this study, we also uncover a useful structural property useful for optimization problems beyond RL.
Autobiography:
Ping-Chun Hsieh is currently an associate professor in the Department of Computer Science at National Yang Ming Chiao Tung University (NYCU). He received his B.S. and M.S. in Electrical Engineering from National Taiwan University in 2011 and 2013, respectively, and his Ph.D. degree in Electrical and Computer Engineering from Texas A&M University (TAMU) in 2018. His research interests include reinforcement learning, multi-armed bandits, and wireless networks. His research received the Best Paper Awards from ACM MobiHoc 2020 and ACM MobiHoc 2017. He is a recipient of Junior Faculty Award (黃培城青年講座) from NYCU, NSTC 2030 Emerging Young Scholar Program (2030新秀學者), MOST Young Scholar Fellowship (愛因斯坦計畫), and the Government Scholarship to Study Abroad from the Ministry of Education, Taiwan.