2024/11/29(Fri.) 14:20 林家祥 教授 國立成功大學電機工程學系 - Unsupervised Hyperspectral Anomaly Detection
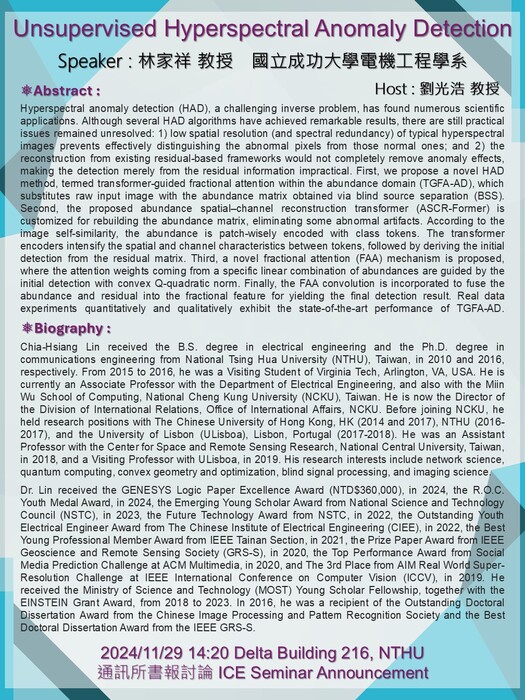
Date & Time:
2024 /11 / 29 (Fri) 14:20 - 16:20
Location:
Delta Building R216, NTHU
Speaker:
林家祥 教授
國立成功大學電機工程學系
Topic:
Unsupervised Hyperspectral Anomaly Detection
Abstract:
Hyperspectral anomaly detection (HAD), a challenging inverse problem, has found numerous scientific applications. Although several HAD algorithms have achieved remarkable results, there are still practical issues remained unresolved: 1) low spatial resolution (and spectral redundancy) of typical hyperspectral images prevents effectively distinguishing the abnormal pixels from those normal ones; and 2) the reconstruction from existing residual-based frameworks would not completely remove anomaly effects, making the detection merely from the residual information impractical. First, we propose a novel HAD method, termed transformer-guided fractional attention within the abundance domain (TGFA-AD), which substitutes raw input image with the abundance matrix obtained via blind source separation (BSS). Second, the proposed abundance spatial–channel reconstruction transformer (ASCR-Former) is customized for rebuilding the abundance matrix, eliminating some abnormal artifacts. According to the image self-similarity, the abundance is patch-wisely encoded with class tokens. The transformer encoders intensify the spatial and channel characteristics between tokens, followed by deriving the initial detection from the residual matrix. Third, a novel fractional attention (FAA) mechanism is proposed, where the attention weights coming from a specific linear combination of abundances are guided by the initial detection with convex Q-quadratic norm. Finally, the FAA convolution is incorporated to fuse the abundance and residual into the fractional feature for yielding the final detection result. Real data experiments quantitatively and qualitatively exhibit the state-of-the-art performance of TGFA-AD.
Autobiography:
Chia-Hsiang Lin received the B.S. degree in electrical engineering and the Ph.D. degree in communications engineering from National Tsing Hua University (NTHU), Taiwan, in 2010 and 2016, respectively. From 2015 to 2016, he was a Visiting Student of Virginia Tech, Arlington, VA, USA. He is currently an Associate Professor with the Department of Electrical Engineering, and also with the Miin Wu School of Computing, National Cheng Kung University (NCKU), Taiwan. He is now the Director of the Division of International Relations, Office of International Affairs, NCKU. Before joining NCKU, he held research positions with The Chinese University of Hong Kong, HK (2014 and 2017), NTHU (2016-2017), and the University of Lisbon (ULisboa), Lisbon, Portugal (2017-2018). He was an Assistant Professor with the Center for Space and Remote Sensing Research, National Central University, Taiwan, in 2018, and a Visiting Professor with ULisboa, in 2019. His research interests include network science, quantum computing, convex geometry and optimization, blind signal processing, and imaging science. Dr. Lin received the GENESYS Logic Paper Excellence Award (NTD$360,000), in 2024, the R.O.C. Youth Medal Award, in 2024, the Emerging Young Scholar Award from National Science and Technology Council (NSTC), in 2023, the Future Technology Award from NSTC, in 2022, the Outstanding Youth Electrical Engineer Award from The Chinese Institute of Electrical Engineering (CIEE), in 2022, the Best Young Professional Member Award from IEEE Tainan Section, in 2021, the Prize Paper Award from IEEE Geoscience and Remote Sensing Society (GRS-S), in 2020, the Top Performance Award from Social Media Prediction Challenge at ACM Multimedia, in 2020, and The 3rd Place from AIM Real World Super-Resolution Challenge at IEEE International Conference on Computer Vision (ICCV), in 2019. He received the Ministry of Science and Technology (MOST) Young Scholar Fellowship, together with the EINSTEIN Grant Award, from 2018 to 2023. In 2016, he was a recipient of the Outstanding Doctoral Dissertation Award from the Chinese Image Processing and Pattern Recognition Society and the Best Doctoral Dissertation Award from the IEEE GRS-S.